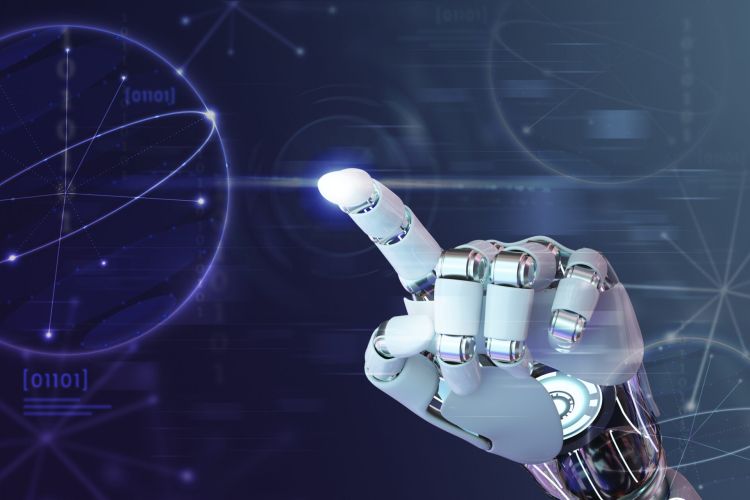
What’s Your Route to Enterprise AI Adoption?
Over the last twenty years, the explosion of data storage and processing needs has prevented this from happening in the business information services sector. It has reportedly virtually destroyed in-house data centers in favor of the cloud.
Now, under the pressure of the latest iteration of the AI hype machine, the explosive interest in machine learning and intelligent process automation is raising the question of where the market for the hot new technology will eventually settle – in external provision or in-house?
In the case of machine learning, the basic model is very different from general cloud provision, as the data is still voluminous, but the technology that enables it is free, relatively easy, and virtually immune to the forces of market capture.
However, a critical difference is the current need for expensive computing resources to develop and update models, a factor that promises to change as lighter machine learning systems such as Apple Core ML create, new players enter the AI ASIC market, and Nvidia’s acquisition of Arm promises to democratize access to GPU-accelerated AI.
Here we’ll look at some of the current indicators that favor an in-house or platform-based approach to enterprise AI development and deployment, given the emerging trends.
Five reasons to develop AI systems in-house
- The best underlying technologies are open source anyway
Over the past decade, the academic origins of open-source and GPU-accelerated machine learning frameworks and libraries have made it almost impossible for well-funded technology giants to lock promising new AI technologies into patent-protected proprietary systems.
This is because almost all foundational developments have been the result of an international collaboration involving a few academic research organizations and government or commercial institutions and because permissive licensing has facilitated this level of global cooperation.
With a few exceptions in the military sector3 and parts of Asia4, publicly funded research is accountable to the public, while commercial attempts to take promising code into private hands would result in a fatal loss of community understanding and development.
Eventually, all of the major technology players were forced to join the open-source AI eco structure in the hope that some other differentiator, such as Microsoft’s capture of the business market, Amazon’s giant consumer reach, or Google’s growing mountain of data, could subsequently deliver unique corporate benefits.
This unprecedented level of technology transparency and openness provides any private commercial project with free, world-class machine learning libraries and frameworks that are not only accepted and well-funded (though not owned) by major technology players but also protected from subsequent revisionist licensing.
- Protecting corporate intellectual property
Most in-house AI projects have a more fragile chance of success than FAANG companies, such as the patentable use concept or the use of internal consumer data – cases where the configuration and development of an AI stack is simply a deployment consideration rather than a value proposition per se.
To avoid encroachment, it may be necessary to tokenize transactions that pass through the cloud infrastructure but retain local control over the central transaction engine.
Where client-side latency is a concern, opaque but functional algorithms based on machine learning techniques can also be deployed, rather than trusting the entire system to the cloud and encrypting or tokenizing returned data for local analysis.
Such hybrid approaches are becoming increasingly common7 amid a growing number of breach reports8 and hacking scandals over the past decade9.
- Data management and compliance controls
The specifics of the raw data for machine learning models are so lost in the learning process that the concern for controlling and managing the raw data for training may seem irrelevant, and shortcuts may be tempting.
However, controversial results from algorithms can lead to an unequivocal conclusion of bias and embarrassing public audits11 of the essential training inputs and techniques used.
Internal systems more easily deter such anomalies once they are identified. This approach ensures that any such impediments to the development of machine learning do not go beyond the terms of cloud AI providers and violate the lattice of various privacy and governance laws that need to be considered when deploying cloud-based AI processing systems.
- AIaaS can be used for rapid prototyping
The opposition between enterprise AI built in-house and cloud-based, or outsourced AI development is not a zero-sum game. The proliferation of open-source libraries and frameworks in the most popular cloud-based AI solutions allows rapid prototyping and experimentation using core technologies that can be transferred in-house once a proof-of-concept is created but is more challenging for an on-premises team to creatively explore on an ad hoc basis.
- High-volume providers are not suited to marginal use cases
Unless the internal project is geared towards the most massive use-cases of external providers, such as computer vision or natural language processing, the deployment, and tooling are likely to be more complex and time-consuming. In addition, they are likely to lack quick-start features, such as applicable pre-trained models, suitable customizable analytical interfaces, or suitable preprocessing pipelines.
Final Thoughts
A data-centric mindset is imperative to successful roadmap completion. Proper enterprise acceleration of maturity for AI adoption means that companies should focus on optimizing their data units and preparing educational initiatives prior to commencing the implementation process. Organizations should also be dynamic in their approach, and be willing to amend their business strategy to incorporate artificial intelligence at every step of the way. This is the essence of a digitally mature AI enterprise.