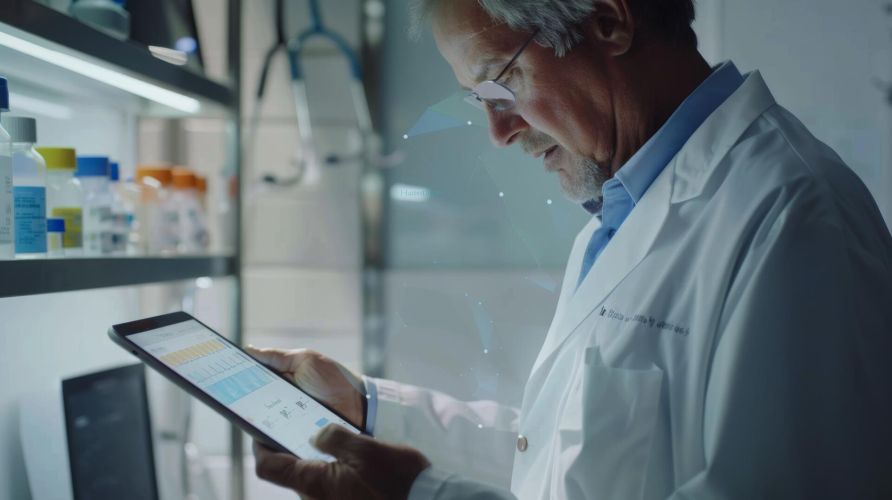
The Future of Data Analytics in Personalized Medicine
Healthcare delivery is undergoing a monumental shift. No longer bound by the one-size-fits-all approach, the medical field is moving toward personalized medicine, where treatment plans are tailored to each patient’s unique characteristics. This transformative approach promises better outcomes, fewer side effects, and more efficient resource use. At the core of this revolution lies data analytics, a tool that processes and interprets vast amounts of information to uncover patterns, predict outcomes, and optimize treatments.
In this article, we delve deep into how data analytics is paving the way for personalized medicine, the challenges that lie ahead, and the exciting innovations that promise to reshape healthcare as we know it.
The Dawn of Personalized Medicine
Personalized medicine, also known as precision medicine, has its roots in the recognition that individuals differ in their genetic makeup, lifestyle, environment, and response to treatments. While traditional medicine applies generalized protocols, personalized medicine uses patient-specific data to:
- Predict Disease Risks: Understanding predispositions to conditions like cancer, diabetes, or cardiovascular disease.
- Guide Treatments: Using genetic and other biological markers to determine the most effective therapies.
- Optimize Drug Use: Tailoring medications to ensure maximum efficacy with minimal side effects.
- Prevent Diseases: Designing lifestyle interventions that mitigate risk factors.
This approach was more theoretical than practical for decades due to technological limitations and data availability. Today, personalized medicine is becoming a reality with advances in computing power, machine learning, and data analytics.
The Key Role of Data Analytics
Data analytics is the backbone of personalized medicine, enabling complex datasets’ integration, processing, and interpretation. Here’s how it is transforming the field:
1. Decoding the Human Genome
Completing the Human Genome Project in 2003 was a watershed moment in medicine. Since then, sequencing technologies have become faster and more affordable, generating a wealth of genetic data. Data analytics is crucial for interpreting this data, identifying gene mutations and patterns linked to diseases, and predicting individual responses to treatment.
Real-World Application:
- BRCA Gene Analysis: Women with mutations in the BRCA1 or BRCA2 genes face significantly higher risks of breast and ovarian cancer. Analytics tools identify these mutations and assess risk levels, enabling early intervention.
Impact:
- Early detection and personalized prevention strategies improve outcomes and lower healthcare costs.
2. Integrating Multimodal Data
Healthcare data comes from diverse sources—genomics, electronic health records (EHRs), wearable devices, imaging studies, and even social determinants of health. Data analytics platforms consolidate these datasets to provide a comprehensive health profile for each patient.
Example:
- Wearable devices like fitness trackers and smartwatches continuously monitor heart rate, sleep patterns, and activity levels. Combining this data with medical histories enables real-time patient health insights.
Impact:
- Through continuous, personalized monitoring, Doctors can predict and prevent adverse events, such as heart attacks.
3. Predictive Modeling and Machine Learning
Machine learning algorithms analyze historical and real-time patient data to predict disease progression and treatment outcomes accurately. This removes much of the guesswork from medical decision-making.
Example:
- In diabetes management, predictive models can forecast blood sugar fluctuations, allowing for proactive diet, exercise, or medication adjustments.
Impact:
- Patients experience fewer complications, and healthcare systems save costs by avoiding hospitalizations.
4. Pharmacogenomics: The Right Drug, The Right Dose
Not everyone responds to medications in the same way. Pharmacogenomics studies the interaction between an individual’s genetics and their response to drugs. Data analytics identifies these relationships, helping doctors prescribe the most effective medication and dosage for each patient.
Example:
- Warfarin, a common blood thinner, requires precise dosing to avoid complications. Genetic testing combined with data analytics ensures accurate dosing for each individual.
Impact:
- Fewer adverse reactions and more effective treatments improve patient safety.
5. Real-Time Monitoring and Personalized Feedback
Wearable and implantable devices generate real-time health data, which analytics tools process to provide immediate feedback. This enables dynamic adjustments to treatment plans based on current conditions.
Example:
- Insulin pumps for diabetics now integrate with continuous glucose monitors (CGMs) to deliver personalized insulin doses.
Impact:
- Patients gain greater control over their conditions, improving quality of life and reducing emergency care visits.
Overcoming Challenges in Personalized Medicine
While the potential of personalized medicine is immense, several challenges must be addressed to fully realize its benefits.
1. Ensuring Data Privacy and Security
Healthcare data is highly sensitive. Breaches can have severe consequences, from identity theft to insurance discrimination. Technologies like blockchain are being explored to manage and share data securely.
Solution:
- Enforce strict compliance with HIPAA (U.S.) and GDPR (EU) regulations.
- Adopt robust encryption and decentralized data-sharing platforms.
2. Breaking Down Data Silos
Data is often trapped in isolated systems, limiting its utility. Interoperability standards and cloud-based solutions are essential for seamless integration.
Example:
- The FHIR (Fast Healthcare Interoperability Resources) standard facilitates data sharing between EHR systems.
3. Addressing Bias in Data
AI and analytics models can inherit biases from training data, leading to inequitable outcomes.
Solution:
- Curate diverse datasets and rigorously test algorithms for fairness.
4. Cost and Accessibility
Sophisticated technologies like genome sequencing remain expensive, making personalized medicine inaccessible for many.
Solution:
- Public-private partnerships and government funding can subsidize costs, ensuring equitable access.
The Future of Data Analytics in Personalized Medicine
Looking ahead, data analytics will continue to revolutionize personalized medicine through the following innovations:
1. AI-Powered Insights
Artificial intelligence will analyze data at unprecedented speeds, uncovering hidden patterns that were previously undetectable.
Example:
- AI-driven tools identifying rare genetic disorders based on subtle anomalies in genomic data.
2. Digital Twins
A digital twin is a virtual patient replica, incorporating their genetic, clinical, and lifestyle data. Doctors can simulate various treatment options on the digital twin to predict real-world outcomes without risk.
Example:
- Testing chemotherapy regimens virtually before administering them to cancer patients.
3. Blockchain for Data Security
Blockchain ensures secure, transparent data-sharing among healthcare stakeholders while preserving patient consent.
Example:
- Decentralized platforms enable patients to control access to their genetic data for research purposes.
4. Population Health Analytics
While personalized medicine focuses on individuals, analytics can also benefit groups by identifying health trends within specific populations.
Example:
- Identifying regions with high rates of chronic disease and implementing targeted interventions.
5. Collaborative Ecosystems
Personalized medicine thrives on collaboration among researchers, clinicians, and tech companies. Shared resources accelerate innovation.
Conclusion: The Road Ahead
Personalized medicine, powered by data analytics, represents a profound leap forward in healthcare. It promises treatments that are more effective, safer, more efficient, and tailored to each individual. However, achieving this vision requires addressing challenges related to privacy, cost, and accessibility.
Integrating advanced analytics, AI, and secure data-sharing technologies will further accelerate progress. As these innovations unfold, the future of healthcare will be defined by precision, prevention, and empowerment, ushering in a new era where the patient is truly at the center of care.
The journey has just begun, but the destination — a healthier, more personalized future — is well within reach.