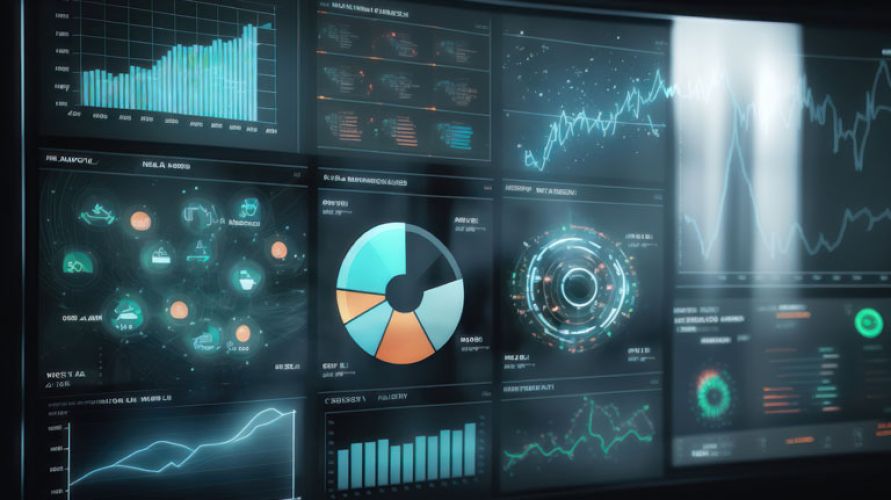
Cognitive Bias in Visual Analytics: A Comprehensive Overview
Visual analytics is an emerging interdisciplinary field that combines visualization, human cognition, and data analysis to help individuals and organizations understand complex datasets. However, like any form of data analysis, visual analytics is susceptible to various cognitive biases, which can greatly affect the interpretation of information. Understanding these biases can provide crucial insights into decision-making processes and aid in producing more reliable and objective results. This article seeks to explore the concept of cognitive bias in visual analytics and outline the most common types of biases, their implications, and ways to mitigate them.
Cognitive Bias: A Definition
Cognitive biases are systematic thinking errors that affect our judgments and decisions. They stem from our brain’s tendency to simplify processing information. When applied to visual analytics, cognitive biases can lead to misinterpretations of data, flawed conclusions, and potential misinformation.
Common Cognitive Biases in Visual Analytics
- Confirmation Bias
This bias occurs when people seek out or interpret data in a way that confirms their pre-existing beliefs or hypotheses while disregarding contradicting evidence. In visual analytics, this can lead to skewed results and biased interpretations of data visualizations.
- Anchoring Bias
Anchoring bias refers to the tendency to rely heavily on the first piece of information (the “anchor”) when making decisions. In visual analytics, this bias could lead to overemphasizing a particular data point or trend that was initially presented, thereby influencing the interpretation of subsequent data.
- Framing Bias
Framing bias is the tendency of people to be influenced by the way information is presented rather than the information itself. For example, a chart or graph that highlights certain data points over others can inadvertently lead viewers to focus more on the highlighted points and ignore other potentially relevant data.
- Apophenia
Apophenia refers to the tendency to perceive patterns or connections in random data. In visual analytics, it can lead to erroneous conclusions and misinterpretations of data visualizations, especially when complex data is involved.
- Availability Heuristic
The availability heuristic is a mental shortcut that relies on immediate examples that come to mind. When interpreting visual data, individuals may place greater importance on information that is readily available or recently encountered, which can lead to an overestimation of certain data points or trends.
The Impact of Cognitive Biases
The impact of cognitive biases in visual analytics is significant. They can lead to skewed perceptions, inaccurate data interpretation, and misinformed decision-making. This affects not only individuals but also organizations and societies at large, particularly when biased visual data analysis is used in critical areas such as policy-making, healthcare, or business strategy.
Mitigating Cognitive Bias in Visual Analytics
Though it’s challenging to completely eliminate cognitive biases, understanding their presence and influence can help mitigate their effects. Here are some strategies:
- Awareness and Training
Training analysts and decision-makers about cognitive biases can create awareness, helping them recognize and correct these biases in their work. Regular training and workshops can be an effective way to continually address this issue.
- Diverse Perspectives
Encouraging diversity in teams can help reduce biases. Different perspectives can lead to a more balanced view of data and help identify potential biases in data analysis and interpretation.
- Data Visualization Best Practices
Follow data visualization best practices to minimize the chances of inducing bias. For example, accurately representing the scale, avoiding misleading colors or designs, and clearly labeling all data points and trends can reduce misinterpretation.
- Peer Review
Incorporate a system of peer review where multiple individuals or teams review the data analysis results. This can help identify any potential biases and improve the overall objectivity of the interpretation.
- Utilizing Automated Tools
Leveraging automated tools and algorithms can help reduce human biases. Such tools can be used to cross-verify the findings and ensure a more objective analysis.
Conclusion
Cognitive biases are a fundamental part of human decision-making, and their impact on visual analytics is substantial. By understanding and identifying these biases, we can mitigate their effects, leading to more accurate interpretations of data visualizations. Through a combination of awareness, diversity, best practices, peer review, and automated tools, we can ensure that visual analytics serves as an objective and powerful tool in the face of complex data. While the journey to unbiased data interpretation may be challenging, it’s an essential step in harnessing the true power of visual analytics.