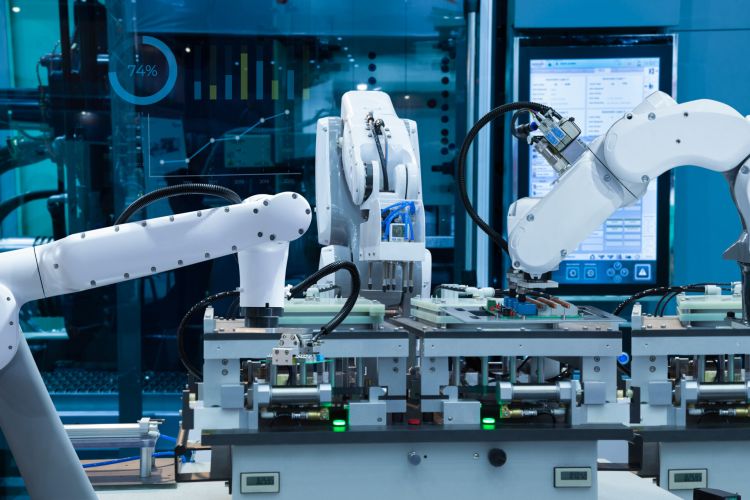
AI Explainability and Adoption in Manufacturing
Artificial Intelligence (AI) is becoming a game-changer in many industries, with manufacturing being one of the sectors at the forefront of this digital transformation. As the fourth industrial revolution, or Industry 4.0, continues to evolve, AI-driven technologies like Machine Learning (ML), Natural Language Processing (NLP), and Robotic Process Automation (RPA) are increasingly implemented to enhance production efficiency, improve quality control, and optimize supply chain management. Despite the promising benefits, a critical factor that still hampers the widespread adoption of AI in manufacturing is the issue of “Explainability.” This article will dive deep into the role of AI in manufacturing, the importance of explainability, and how overcoming this barrier can facilitate greater AI adoption.
The Role of AI in Manufacturing
AI in manufacturing goes far beyond the simple automation of tasks. It involves intelligent machines that can predict outcomes, make decisions, and learn from their mistakes. Advanced algorithms can analyze enormous volumes of data to spot trends, identify bottlenecks, and make predictions about future performance.
For example, predictive maintenance powered by AI can analyze data from equipment sensors to predict machine failure before it occurs, thus saving time and reducing costs. Similarly, AI-based quality control systems can identify defects in products faster and more accurately than human inspectors. In the supply chain, AI can forecast demand, optimize logistics, and streamline inventory management, making the whole process more efficient and cost-effective.
The Importance of AI Explainability
Despite the myriad of opportunities, the adoption of AI in manufacturing is not without its challenges. One significant hurdle is the issue of explainability, also known as the ‘black box’ problem. AI and ML algorithms, particularly those based on deep learning, often produce results without a clear explanation of how they arrived at their conclusions.
Explainability is vital in the manufacturing context for several reasons.
Trust: For the staff to trust AI predictions and decisions, they need to understand how the AI system arrived at its conclusion.
Accountability: When things go wrong, as they inevitably will at times, it’s crucial to determine the cause of the problem to prevent its recurrence. If an AI system makes a decision that leads to a production defect or other significant issues, manufacturers need to be able to dissect the decision-making process.
Regulatory Compliance: Certain industries have stringent regulations requiring companies to explain their decision-making processes, especially in areas affecting safety, quality, and environmental impact.
Enhancing AI Explainability
Addressing the issue of explainability involves making AI decisions more transparent, understandable, and interpretable. There are two primary approaches to this: post-hoc explainability and interpretable models.
Post-hoc explainability involves explaining an AI decision after it has been made. Techniques used include Local Interpretable Model-Agnostic Explanations (LIME), which creates simple, local models to explain how the AI system made its decision in individual cases, and Shapley Additive Explanations (SHAP), which attributes the contribution of each feature to the final decision.
Interpretable models, on the other hand, are designed to be understandable from the outset. These include decision trees and rule-based systems that provide a clear, if simplified, map of how they reach decisions.
Facilitating AI Adoption in Manufacturing
By enhancing the explainability of AI systems, manufacturers can build trust among their workforce, maintain accountability, and meet regulatory requirements. This will greatly facilitate AI adoption in the industry. Here are some strategies for doing this:
Educating the Workforce: As AI systems become more prevalent, it’s crucial that employees at all levels of the organization have a basic understanding of AI. This does not mean that every worker needs to become an AI specialist, but they should have enough knowledge to understand how AI impacts their role and the overall manufacturing process.
Working with Transparent AI Vendors: Manufacturers should seek to work with AI vendors that prioritize transparency and explainability. Vendors should be able to explain in understandable terms how their system works and how it makes decisions.
Adopting an Incremental Approach: Instead of trying to implement AI across the entire manufacturing process at once, it can be beneficial to start with one area, such as predictive maintenance or quality control. This allows employees to gradually become familiar with AI systems and their benefits.
Conclusion
By prioritizing explainability, manufacturers can begin to disassemble the ‘black box’ of AI, uncovering the logic behind its decision-making processes. This increased transparency does more than just satisfy intellectual curiosity; it builds a foundation of trust between the AI systems and the human workforce. In an industry where precision, reliability, and repeatability are key, this trust forms an integral part of a seamless AI integration.
While AI’s adoption in manufacturing is laced with challenges, focusing on explainability can turn these challenges into opportunities for growth, innovation, and improved efficiency. By demystifying AI, we open doors to increased adoption rates, creating a future where humans and AI work hand in hand, harnessing the power of intelligent automation to drive the manufacturing industry forward. The journey to AI adoption may be complex, but with explainability at its core, it is a journey that promises a paradigm shift in the world of manufacturing.