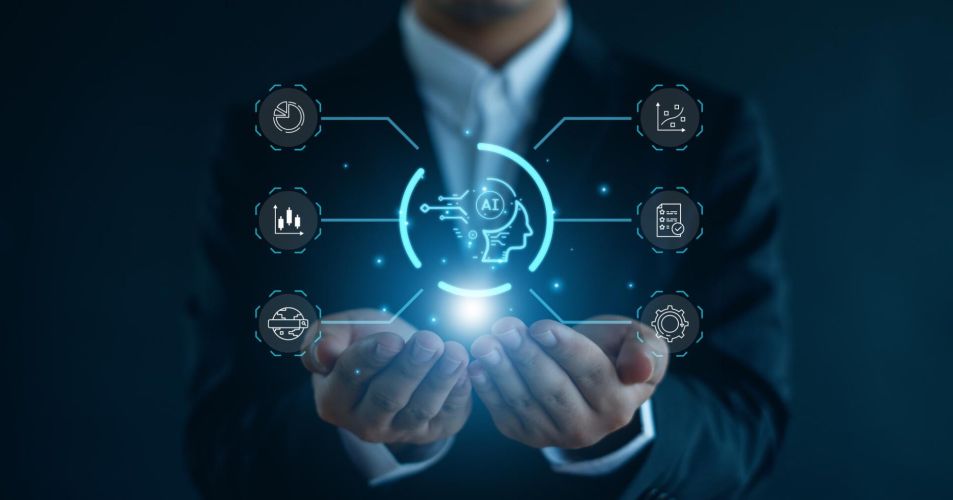
AI and Machine Learning in Business Decision-Making: A Comprehensive Exploration
When businesses are inundated with data, the ability to process, analyze, and act on this information has become a cornerstone of success. The advent of artificial intelligence (AI) and machine learning (ML) has provided enterprises with the tools to extract actionable insights from massive datasets, enabling smarter and more agile decision-making. No longer confined to tech giants, these technologies are being adopted across industries, from retail to finance, healthcare to manufacturing.
This article delves deeply into how AI and ML reshape business decision-making processes, offering detailed examples, case studies, and insights into the benefits, challenges, and future trends.
The Evolution of Business Decision-Making
Traditionally, business decisions relied on historical data, intuition, and human expertise. While these methods are still relevant, they often fall short in today’s fast-paced, globalized, and highly competitive environment. AI and ML bridge these gaps by enabling:
- Speed: Decisions can be made in real time using live data.
- Scalability: AI systems can process exponentially larger datasets than human analysts.
- Precision: ML algorithms identify nuanced patterns that human intuition might overlook.
- Automation: Routine decision-making processes can be automated, freeing up human resources for strategic initiatives.
For example, a marketing team can use AI tools to decide the best time to launch a campaign, while predictive models help the finance department forecast revenue trends.
Key Areas of AI and ML Integration in Decision-Making
AI and ML enhance decision-making across multiple domains within a business. Below, we explore some of the most impactful areas.
1. Predictive Analytics
Predictive analytics employs historical data and ML models to predict future outcomes. This capability is widely used across industries to anticipate customer behaviors, market trends, and operational risks.
Example in Retail:
Walmart uses predictive analytics to anticipate inventory needs. By analyzing sales trends, weather forecasts, and regional demand, Walmart ensures its shelves are stocked with the right products at the right time.
Example in Healthcare:
Predictive analytics helps healthcare providers identify patients at risk of chronic illnesses, enabling preventive care and reducing hospital readmissions.
2. Personalized Customer Experiences
AI-powered systems analyze customer data to tailor products, services, and communications. Personalization not only enhances customer satisfaction but also drives sales and loyalty.
Example:
E-commerce platforms like Amazon recommend products based on browsing history, purchase behavior, and demographic data. These recommendations account for a significant portion of Amazon’s revenue.
3. Dynamic Pricing Strategies
Dynamic pricing involves adjusting prices in response to demand, competitor pricing, and other market variables. AI systems can monitor and adjust prices in real time to maximize revenue.
Example:
Airlines and hotels use dynamic pricing algorithms to optimize ticket and room rates. For instance, during peak travel seasons, prices rise based on high demand, while discounts are offered during off-peak times.
4. Fraud Detection and Risk Management
AI and ML excel in identifying irregular patterns in financial transactions, employee behaviors, or supply chain activities, which may indicate fraud or risk.
Example:
PayPal’s fraud detection system uses ML to analyze millions of transactions in real time. It identifies anomalies, such as unexpected spending patterns, and flags potential fraudulent activities.
5. Operational Efficiency
AI-driven tools optimize operations by automating repetitive tasks, streamlining workflows, and predicting maintenance needs.
Example in Manufacturing:
General Electric (GE) uses predictive maintenance to minimize downtime. Sensors on machinery collect performance data, and AI analyzes this data to predict when equipment will require maintenance.
Case Studies: Success Stories Across Industries
To illustrate the transformative power of AI and ML, let’s dive into detailed case studies from various sectors.
Case Study 1: Coca-Cola – Enhancing Customer Engagement
Coca-Cola employs AI to improve its marketing and product development. Using sentiment analysis on social media, the company gathers customer opinions about its products. This data-driven approach has led to the creation of new beverages and targeted marketing campaigns.
Case Study 2: IBM Watson – Revolutionizing Healthcare
IBM Watson’s AI system assists oncologists by analyzing vast medical datasets and providing treatment recommendations. Watson cross-references patient data with medical journals, clinical trials, and historical case studies to support complex medical decisions.
Case Study 3: Spotify – Redefining Music Discovery
Spotify uses ML algorithms to analyze user preferences, creating highly personalized playlists. Features like “Discover Weekly” and “Release Radar” are powered by AI, driving user engagement and retention.
Case Study 4: Tesla – Autonomous Driving Decisions
Tesla’s AI-powered autopilot system uses ML to make real-time driving decisions. The system adjusts speed, lane positioning, and braking to ensure safe and efficient navigation by analyzing data from cameras, sensors, and GPS.
AI and ML in Small and Medium-Sized Enterprises (SMEs)
While large corporations are at the forefront of AI adoption, SMEs increasingly leverage these technologies. Cloud-based AI tools, such as Google Cloud AI and AWS AI, make it affordable for smaller businesses to integrate ML into their operations.
Example:
A small online retailer might use AI to recommend products or forecast inventory needs without building a complex in-house system.
Advantages of AI and ML in Decision-Making
The integration of AI and ML offers transformative benefits:
- Enhanced Decision Accuracy: Algorithms analyze data objectively, reducing human error.
- Increased Speed: AI systems process data and generate insights in real time.
- Resource Optimization: Automating decision-making frees employees for higher-value tasks.
- Competitive Advantage: Companies that leverage AI gain a data-driven edge over competitors.
Challenges and Ethical Considerations
Despite their benefits, AI and ML pose challenges that businesses must address:
- Data Privacy: Collecting and analyzing customer data raises privacy concerns.
- Algorithmic Bias: ML models can inherit biases present in the training data.
- Transparency: Decision-making processes powered by AI often lack explainability, leading to mistrust.
- Cost and Expertise: Implementing AI requires significant investments in technology and skilled personnel.
- Regulatory Compliance: Businesses must navigate a complex landscape of regulations regarding AI use.
Example:
In 2019, Apple faced criticism for its credit card algorithm, allegedly discriminating against women. The incident highlighted the importance of fairness and transparency in AI-driven decision-making.
Future Trends in AI and ML for Decision-Making
As technology advances, AI and ML will redefine how businesses make decisions. Emerging trends include:
- Explainable AI (XAI): Ensuring transparency by making AI decision-making processes understandable to humans.
- AI-Driven Collaboration: Combining AI insights with human expertise to enhance decision quality.
- Hyper-Automation: Automating end-to-end business processes using AI, robotics, and IoT.
- Decentralized AI: Integrating AI across distributed systems for greater scalability and resilience.
Conclusion
AI and machine learning are no longer futuristic concepts but indispensable tools in modern business decision-making. By harnessing the power of data, businesses can predict trends, personalize customer experiences, optimize operations, and mitigate risks. However, the road to AI integration is not without challenges. Companies must navigate ethical concerns, data quality issues, and high implementation costs.
As more businesses adopt these technologies, the competitive landscape will evolve. Organizations that embrace AI and ML strategically will enhance their decision-making capabilities and drive innovation, growth, and resilience in an ever-changing market. AI and ML are more than just tools — they are catalysts for a smarter, more agile future.